Beatriz Peñalver Bernabé
Beatriz Peñalver Bernabé
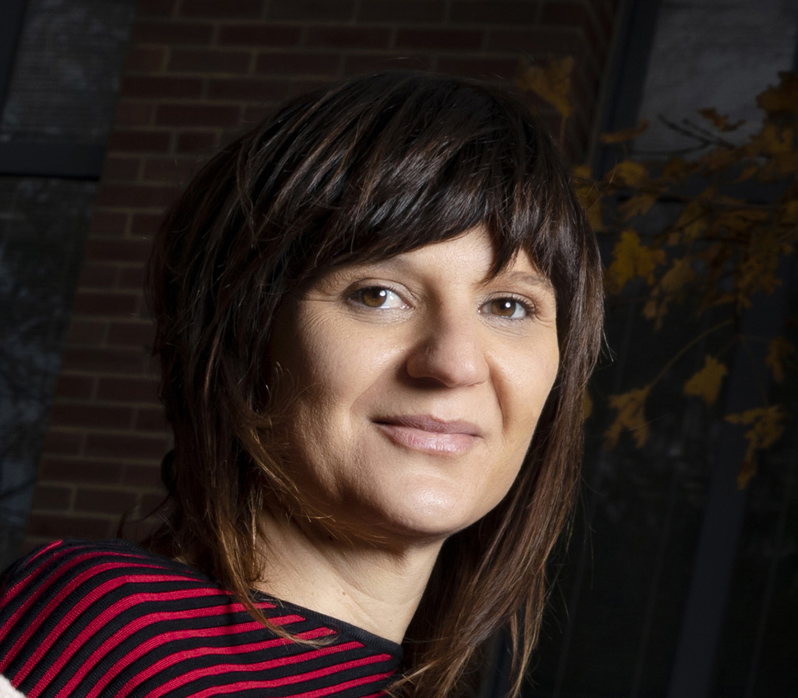
Biomedical Engineering Faculty
Dr. Beatriz Peñalver Bernabé, Ph.D.
Profile
I am a chemical and biological engineer focused on understanding and optimizing complex dynamic systems through a wide array of mathematical, statistical, computational and network approaches. Broadly, I have a great interest in dynamic biological processes, especially those with applications in women’s health. During my 8 years working in the polymeric industry, I led several high-profile research and development projects that rendered multiple patents. I then returned to academia to work in applications for translational and regenerative medicine, with emphasis in women reproduction biology. Since 2009, I have been focused on developing statistical and computational platforms (machine learning) to analyze the evolution and treatment of breast cancer and ovarian follicle development in vivo and in vitro for fertility preservation. In all those projects, I have been collaborating with highly inter-disciplinary teams of doctors and clinicians, experimentalists, statisticians and computational experts from the US and abroad.
In 2016, I started a new line of research to study host-microbial interactions in depression during pregnancy and postpartum. We have several ongoing projects in which we recruit pregnant individuals from the outpatient clinics from UIC Hospital that serve mostly serve at UIC are women of color and we follow then after postpartum. We aim to understand the pathobiology of depression during the perinatal time using a whole-person approach through the integration of multiple omics methods (e.g., metagenomics, non-targeted metabolomics) with social, physical (e.g., electroencephalograms) and psychological measurements (e.g., diet, depression symptoms). We are also using machine learning approaches to take advantage of the wealth of information available in Electronical Medical Records to diagnose and predict depression during pregnancy and postpartum.
Description
Please note: prior computer programming experience in R, Python or MATLAB is a plus!
1) Mood Disorders during Pregnancy and Postpartum
It is estimated that approximately 10-20% of women experience depression during pregnancy and postpartum. Untreated depression during pregnancy increases the risk of preterm birth, low birth weight, and intrauterine growth restriction, all of which have life-long consequences for infants later in their lives. Our current knowledge of the pathobiology of depression during pregnancy is limited by an incomplete understanding of the biological mechanisms contributing to it. The gut microbiota (i.e., bacteria, archaea, fungi, protists and viruses) and mental health are linked through the gut-brain axis, which mediates the complex, bidirectional interactions between intestinal bacterial communities and neurological, immunological and endocrinological processes. The microbiome can be manipulated (e.g., antibiotics, prebiotics, probiotics, diet and exercise) making it a useful target for the development of new therapeutics that women may perceived more natural and thus increase the intake and adherence of medication to treat mood disorders in expecting mothers. Our group is exploring the complex and dynamic interactions between depression during pregnancy, the maternal microbiome and the maternal neurological, immunological and endocrinal systems by their integration using advanced statistical and machine learning approaches, Systems Biology.
Note: R and Python is a plus for this project.
2) Ovarian Follicle Development in Humans
Tremendous advances have been made in the last 40 years to understand mammalian reproductive biology and in vitro fertilization (IVF) is one of the most remarkable outcomes. Reliably producing a competent XX germ cell—an oocyte—that can be fertilized entails a deeper comprehension of ovarian follicle maturation, which is a very complex process that includes meiotic maturation of the XX gamete, the oocyte, together with the mitotic divisions of the hormone-producing somatic cells. During ovarian follicle maturation, a primordial follicle that is composed from a handful of cells, has to grow into an antral follicle, 10 times larger, in order to attain a competent oocyte.
Ovarian follicle maturation requires highly orchestrated paracrine, autocrine, endocrine and juxtacrine signaling that has to occur between the oocyte and the supporting somatic cells, granulosa and theca cells. Additionally, ovarian follicle development is not possible without the adequate supply of nutrients to the oocyte, as the oocyte must produce and store all the components required to support multiple divisions after fertilization. Moreover, ovarian follicle maturation metabolism and signaling are largely unknown, especially at the early stages of follicle development. Therefore, we are developing intra-cellular and inter-cellular signaling networks and context-specific metabolic models during ovarian follicle maturation in vivo using single-cell RNA-seq and mathematical modelling to identify possible growth factors and metabolites to add to the culture media to grow human primordial follicles into mature oocytes in vitro.
Note: MATLAB is a plus for this project.